What Generative AI and Large Language Models Mean for Customer Service & Machine Translation
With the meteoric rise of generative AI in recent months, Language I/O has started to receive questions from customers and prospects regarding the role of our machine translation platform in the era of large language models (LLMs). Here’s everything you need to know about the short- and long-term impact of generative AI on machine translation.
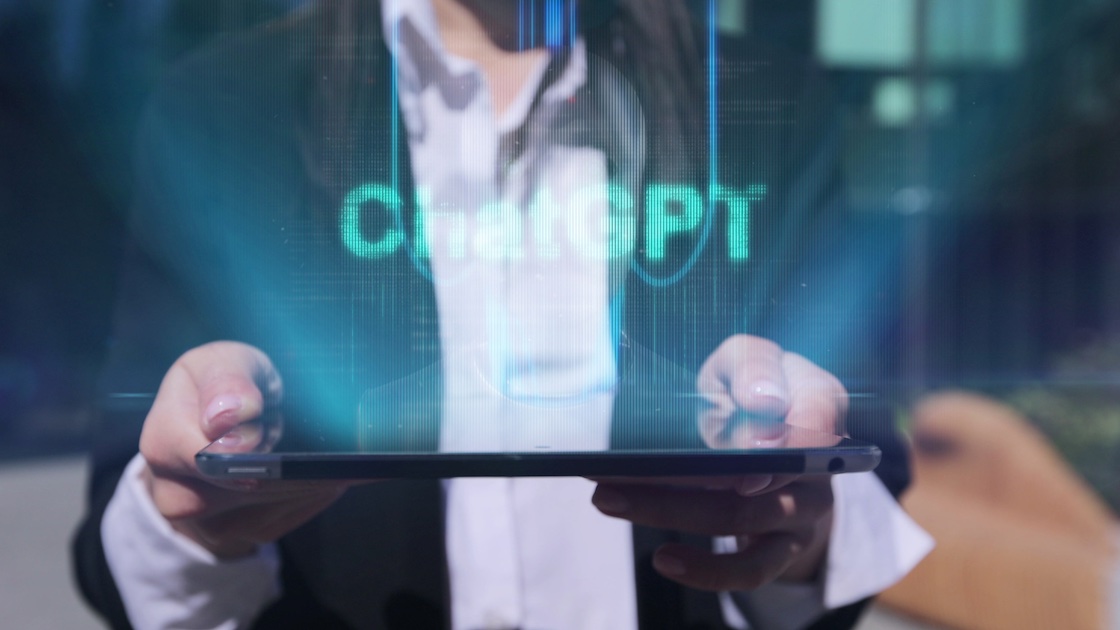
Subscribe to our newsletter
Share
With the meteoric rise of generative AI in recent months, Language I/O has started to receive questions from customers and prospects regarding the role of our machine translation platform in the era of large language models (LLMs). Questions include:
- Will our tech still be relevant if LLMs such as ChatGPT and Google Bard begin powering in-language chatbots for customer support?
- Why would machine translation (MT) technology be necessary when an LLM such as ChatGPT is fluent in every language?
- What is Language I/O doing in response to the rise of LLMs?
To answer these questions, let’s first look at the definition of a large language model and related questions.
What is a large language model?
Large language models (LLMs) are computer programs that have the ability to read and interpret human language. Sophisticated LLMs, like ChatGPT, can understand not only the meaning of language but also its intent, and produce responses that address that intent appropriately. To function like this, LLMs are trained on a colossal amount of data, which enables them to understand the patterns of human language and how it is used for communication.
What is an example of a large language model?
Perhaps the most recognizable example of a large language model is GPT-3.5, which the freely available public version of ChatGPT uses. Prior to the release of ChatGPT, GPT-3.5 was trained on a massive corpus of publicly available Internet data.
We’ll come back to that “publicly available” descriptor and why it’s important later on.
How does generative AI impact customer service and machine translation?
When evaluating how generative AI may impact the language translation industry as we know it, the answer requires two perspectives. One takes into account the short-term impact and considerations, while the other looks at the long-term/future implications.
Let’s start with the short-term considerations.
Today, using generative AI tools like ChatGPT for machine translation is not a viable solution for the following reasons:
• Bias & Inaccuracies: Because ChatGPT is trained on the public Internet, which is full of human bias and misinformation, responses from ChatGPT in turn contain all sorts of inaccuracies and bias. At best this results in confusion. At worst use of ChatGPT responses can have serious implications, such as when ChatGPT invented allegations against a lawyer earlier this year. We can expect ChatGPT and other LLMs will be struggling with this issue for years to come, meaning that LLM-powered chatbot sessions will continue to require human supervision and manual improvements.
• Security: In the short term, enterprises are raising very serious concerns about the security of personal data that is passed into these LLMs. Even secure, enterprise versions of ChatGPT will not agree to zero-trace policies, meaning that ChatGPT will continue to store content that is passed through its engine, even once that conversation is complete. This is why Samsung employees were in hot water when they provided confidential information to ChatGPT.
• Gated Content: While the output of LLMs sounds fluent—even human—across many languages, generative AI programs such as ChatGPT or Google Bard run into issues when it comes to what is called gated content. Gated content, as its name might suggest, is anything that is locked behind some kind of gate, such as a login. While marketers know gated content to mean pieces of content that require web visitors to fill out a form in order to access, this term also applies to any information that is not on the publicly accessible Internet. The world’s leading LLMs have been trained solely on the public Internet, meaning the chatbots trained on them alone are unable to answer customer support questions regarding that inaccessible content. To put it simply: LLMs can’t learn from data they can’t access.
The Future of Language-specific Chatbots in Customer Support
Now we will delve deeper into what we can expect from the future of LLMs for customer support. While we’ve covered a number of issues with present-day generative AI and LLMs, we can reasonably expect that these areas of concern will be resolved within the next 5-10 years, if not sooner. With that in mind, it is likely that language-specific chatbots will see significant use, which could potentially negate the need for human support agents or the need for machine translation services in the case that LLMs theoretically speak every language at the same level as a native-speaking human.
In order to accomplish this, brands will need to adapt LLMs by feeding them gated content, such as knowledge base articles and other such proprietary information. To do this even in just one language requires a large corpus of data. While the relevant data set does not have to be as large as the entirety of the Internet, it is still a massive amount of data.
Just as companies today struggle to generate the thousands of intents and utterances needed to properly train an AI-powered chatbot in a single language, it is unlikely that the vast majority of brands in the future will have the resources or internal subject matter expertise to fine-tune LLMs across 10, 20, or even more languages.
The Importance of Training LLMs on Specialized Content (In Every Language)
To explain why adapting LLMs via gated content is necessary, let’s look at some highly specialized content that ChatGPT is unable to translate today, even when provided with the missing context. When we say specialized content, we mean any type of company- or industry-specific technical jargon, invented terminology (such as gaming/character names), recently evolved slang, or even normal words that simply require a specific translation dependent upon context.
We’ll begin with an example from the gaming world. One of Language I/O’s customers received the following support request from a player in Beijing. It’s a great example because it not only includes recently invented slang, but it also uses terms that were specifically invented for Magic the Gathering (MTG). In this customer support request, which is in Chinese, the bolded words are the ‘problematic’ terms and phrases.
我有张毁世奥札奇牌,带歼灭关键字动作。但老是不触发。瞎菜了。能帮我解决这个问题吗?gkd。我的电邮[email protected],我的DCI号是9783472952。
Below is the way MTG needs to have their game-specific terms translated between Chinese and English. We’ve also included the recently-invented Beijing slang terms.
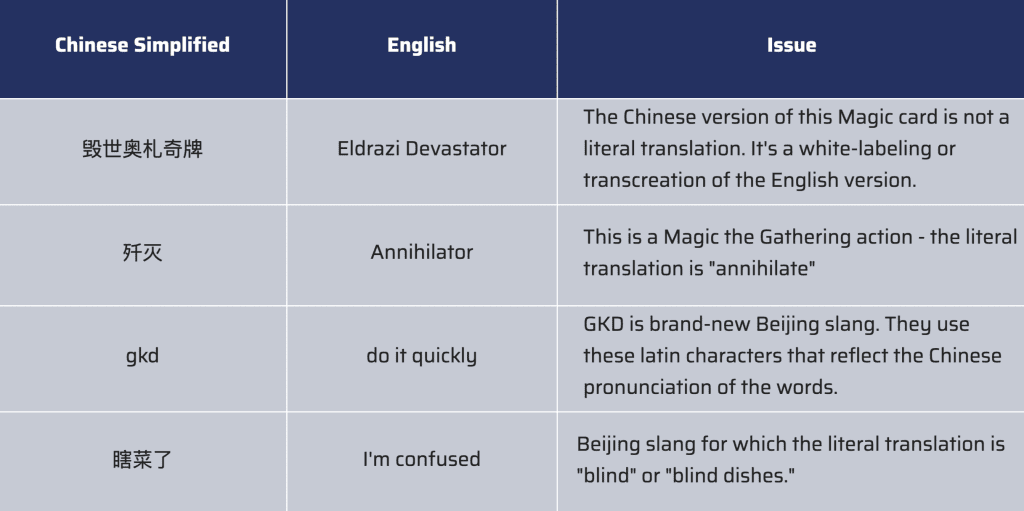
We attempted to translate this Chinese support request into English through ChatGPT, and added the context that this is related to MTG in an attempt to assist the translation:
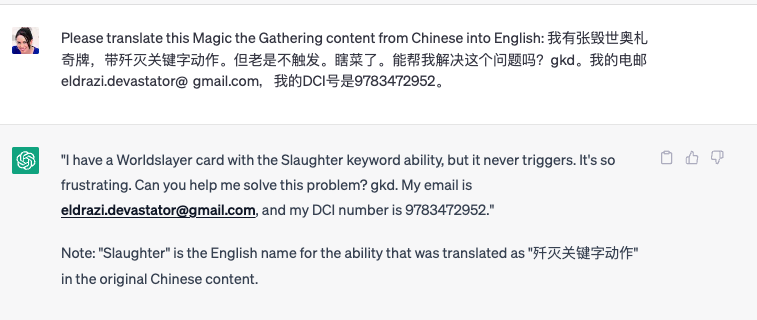
None of these ‘problematic’ terms were properly translated by ChatGPT:
- “毁世奥札奇牌” (Eldrazi Devastator) was translated as “Worldslayer”
- “歼灭” (Annihilator) was translated as “Slaughter”
- “瞎菜了” (I’m confused) was translated as “it’s so frustrating”
- “gkd” (slang for “do it quickly”) was not translated at all
If an MTG support agent is asked about a Worldslayer card with the Slaughter keyword action, they will be baffled. To ChatGPT’s credit, “it’s so frustrating” isn’t a terrible translation, but an English-speaking support agent would have no idea what “gkd” means.
Because some of this information is accessible on the Internet, ChatGPT may eventually be able to accurately translate some of the slang or MTG card names. But bear in mind that LLMs take time to pick up on the new content. The “P” in GPT, for example, stands for “pre-trained.” These models aren’t instantly detecting every new piece of information across the Internet, and so a company leveraging an LLM won’t automatically see new information reflected in its chatbot content. A lag of even a couple of weeks could result in a poor customer experience and lost revenue.
Properly Translating Specialized Content
Keeping with our Eldrazi Devastator example, the below screenshot demonstrates how Language I/O’s technology translates that same gamer’s query from Chinese to English. Our platform first selects the best engine for fluency between Chinese and English, and then instructs that engine in real time how the brand requires any specialized terminology to be translated (or, in some cases, not translated).
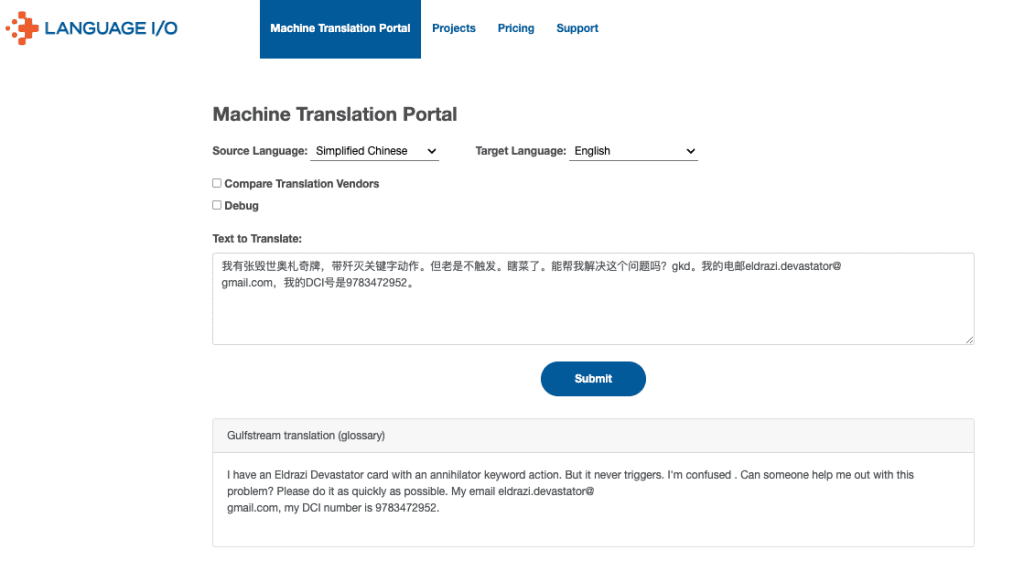
Because our technology recognizes ‘problematic’ terminology and how to properly translate it, the resulting translation is easy for an MTG support agent to process and respond to.
Our tech does this by referencing an internal glossary of specialized terminology, which dictates how each Language I/O customer wants specific terms to be handled when passed through a translation engine. The terms in each customer’s glossary are directly provided by the brand (this is usually the case for organizations with an existing localization team) and/or detected by our self-improving glossary (SIGLO) technology, which is trained to recognize terms specific to an individual domain (such as a company or a specific division of that company) and recommend those terms be added to the glossary.
This glossary layer is important not just for gated content, but also for generic words that require context in order to understand their meaning. Here is an example of a normal English word — “player” — that ChatGPT does not translate properly no matter how much context it is provided.
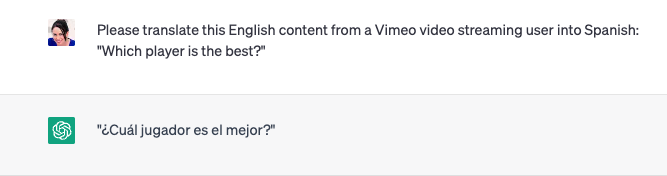
The word “player,” when translated into the context of a video streaming website, should be translated into Spanish as “reproductor.” Here, we can see ChatGPT, even when given information about the use case, still translates “player” into “jugador,” which refers to a sports player (or, athlete). That translation may work for our online gambling clients, for whom “player” always refers to an athlete on which customers are placing a bet, but it doesn’t work here.
Within a video streaming company’s Language I/O account, the correct translation of “reproductor” is used:
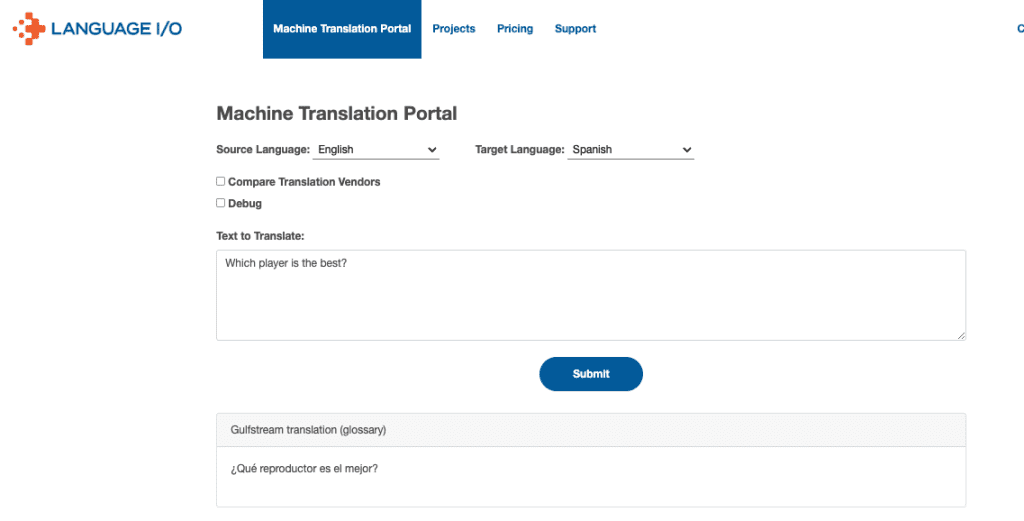
Augmenting Generative AI Outputs with Language I/O
There is no doubt that the rise of LLMs and generative AI chatbots will impact the need for native-speaking customer service agents and machine translation use cases across the board. But even as language-specific LLMs rise in prominence, the need for industry- or brand-specific translations will remain.
Rather than organizations having to take on the massive lift of fine-tuning an LLM for each language they support, a far more palatable and realistic option for the vast majority of companies is to fine-tune an LLM in one language (whichever they use the most) and then use Language I/O to translate those outputs into other languages. Not only does this solve the issues of gated content and domain-specific translations, but it also provides these benefits directly into each organization’s CRM, such as Salesforce for Zendesk, via native integration.
To learn more about Language I/O’s real-time translation for customer support use cases and more, reach out to us or request a demo.