Harnessing the Power of Multiple Translation Engines: A Guide to MT Aggregation Layers
Explore why using multiple translation engines for real-time business conversations is so powerful, while also learning the three critical considerations to look for in your aggregation layer.
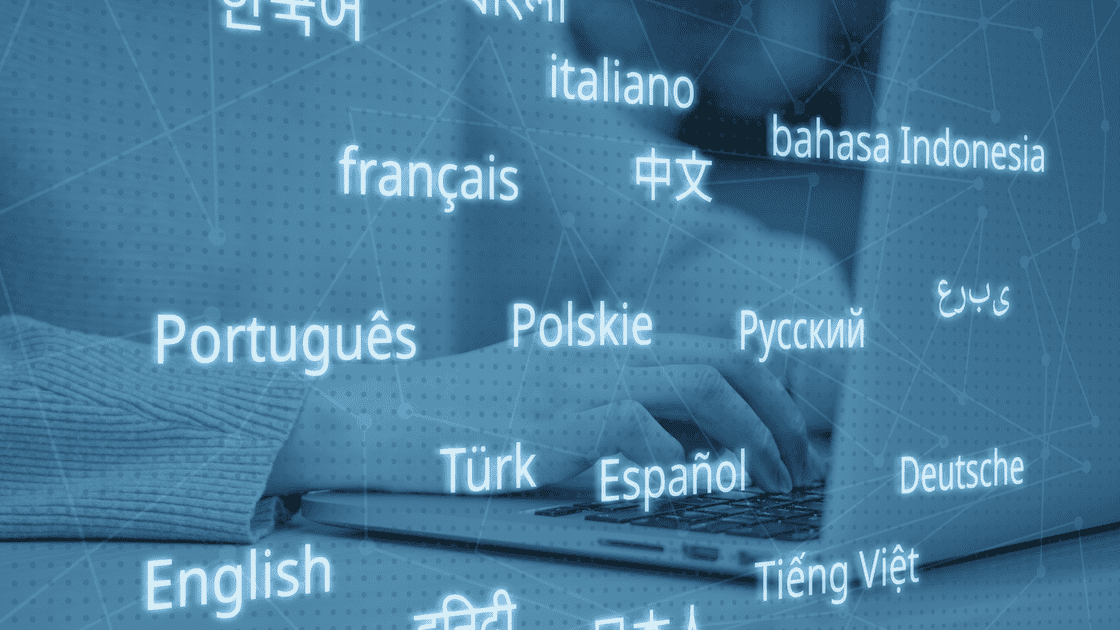
Subscribe to our newsletter
Share
As use of machine translation for internal and external business communication continues to grow, many multinational brands are wisely turning to technology that leverages multiple translation (MT) engines rather than relying on just one engine.
Why is having multiple MT engines at your disposal is better than relying upon just one? The main reason is language proficiency. MT engines generally don’t excel at accurately translating every language that they enable users to work with. For example, Google Translate is generally considered to be proficient when translating languages such as German, French, and Spanish, among others, but struggles with languages such as Korean and Japanese.
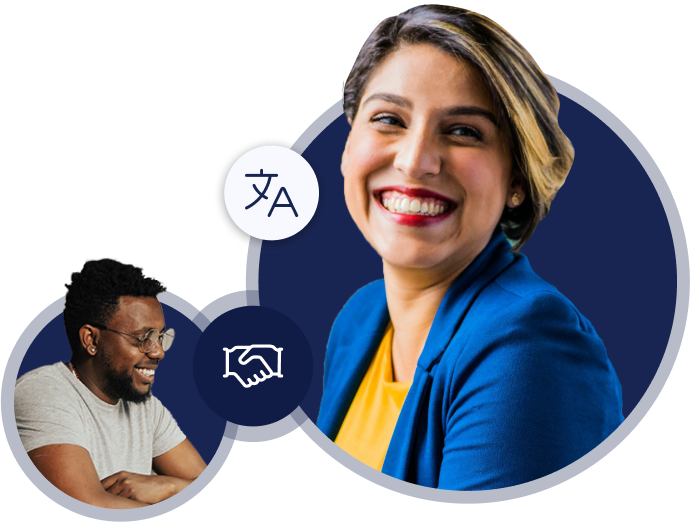
By using multiple engines, a translation provider can toggle between the various engines to use the best one for the language pair in question. A conversation from Spanish to English may be translated via Google Translate or DeepL, but a conversation between a Korean speaker and a Chinese speaker is better served by Papago. And as large language models (LLMs) or generative AI platforms demonstrate their translation fluency as well as increased privacy for business use cases, such as Google’s PaLM, these will be added to the mix as well.
Integrating with multiple MT engines thus allows brands to leverage the strength of each one, which bolsters translation quality and overall accuracy.
However, not all MT aggregation layers are equally viable for brands. Here are the key characteristics that you should consider when looking for a technology that aggregates MT engines.
Security
One of the most critical aspects to consider is the security of the engines included in the MT aggregation layer, also known as a machine translation hub. In today’s high-alert environment, data privacy and protection is paramount. Because real-time communication often contains sensitive, personally identifiable data—think of customer support chat messages that might contain a customer’s real name, address, or even bank account information—it’s crucial to ensure any technology that processes real-time communication is secure.
When considering an MT aggregation layer, brands should determine whether any of the engines within the layer store content or personally identifiable information (PII). Storing such data poses not only a security risk but also potential non-compliance with data protection regulations like the GDPR or CCPA.
Very few machine translation hubs take privacy to the level that Language I/O takes it with its zero-trace platform. The Language I/O machine translation hub only integrates with MT engines that agree in writing not to store any of the content that is passed to them for translation. Neither does the native Language I/O platform store, log or in any way hold onto the content that is passed in for translation, or the translated version. Instead, the Language I/O system operates in a stateless mode where translation requests and their results are immediately discarded after use. Brands should prioritize these kinds of systems to maintain the privacy of their users and adhere to regulatory guidelines.
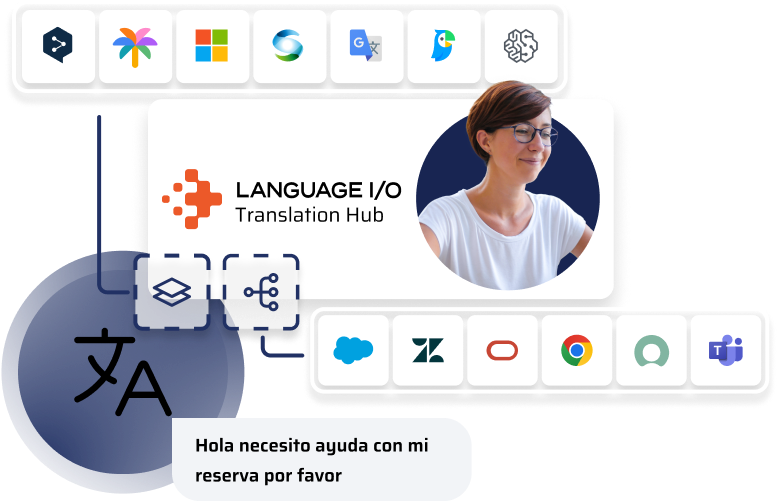
Automation
In addition to security, an effective MT aggregation layer should also have high-quality automation capabilities. The automation should be sophisticated enough to intelligently select the most suitable translation engine for each translation task without requiring the user to do so manually. This not only improves efficiency and speed, but also ensures the best possible translation quality for each language pair.
This is where machine learning comes into play. Advanced MT aggregation layers such as that built by Language I/O, use artificial intelligence to assess each engine’s strengths and weaknesses in translating specific language pairs. The system then automatically selects the engine that is most likely to provide the most accurate translation for the current task.
For example, a sophisticated aggregator such as Language I/O may recognize that for Spanish to English translations, DeepL provides superior results and will default to this engine for such tasks. Alternatively, for Korean to Chinese translations, the system might determine that Papago is the optimal choice and automatically select it.
Integration
Last, but certainly not least, is integration. For real-time, multilingual communication to be truly effective, it needs to integrate seamlessly with the tools your teams are already using. If an MT aggregation layer requires significant changes to existing workflows or software, it can add unnecessary complexity and hinder adoption.
When considering different MT aggregation layers, look for options that are designed to work with your existing communication tools—such as Salesforce, Zendesk, ServiceNow, or Oracle. These systems should have straightforward integration processes and perhaps even provide browser plugins or APIs to simplify setup and configuration.
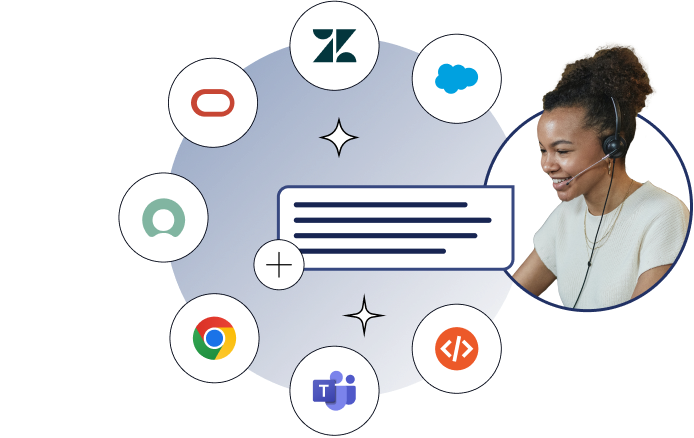
In conclusion, while integrating multiple MT engines can dramatically improve the accuracy of real-time machine translations, it’s important for users to carefully evaluate MT aggregation layers. Paying attention to these three key factors – security, automation, and integration – will help ensure that the technology you select is not only efficient and effective, but also secure and easy to use.